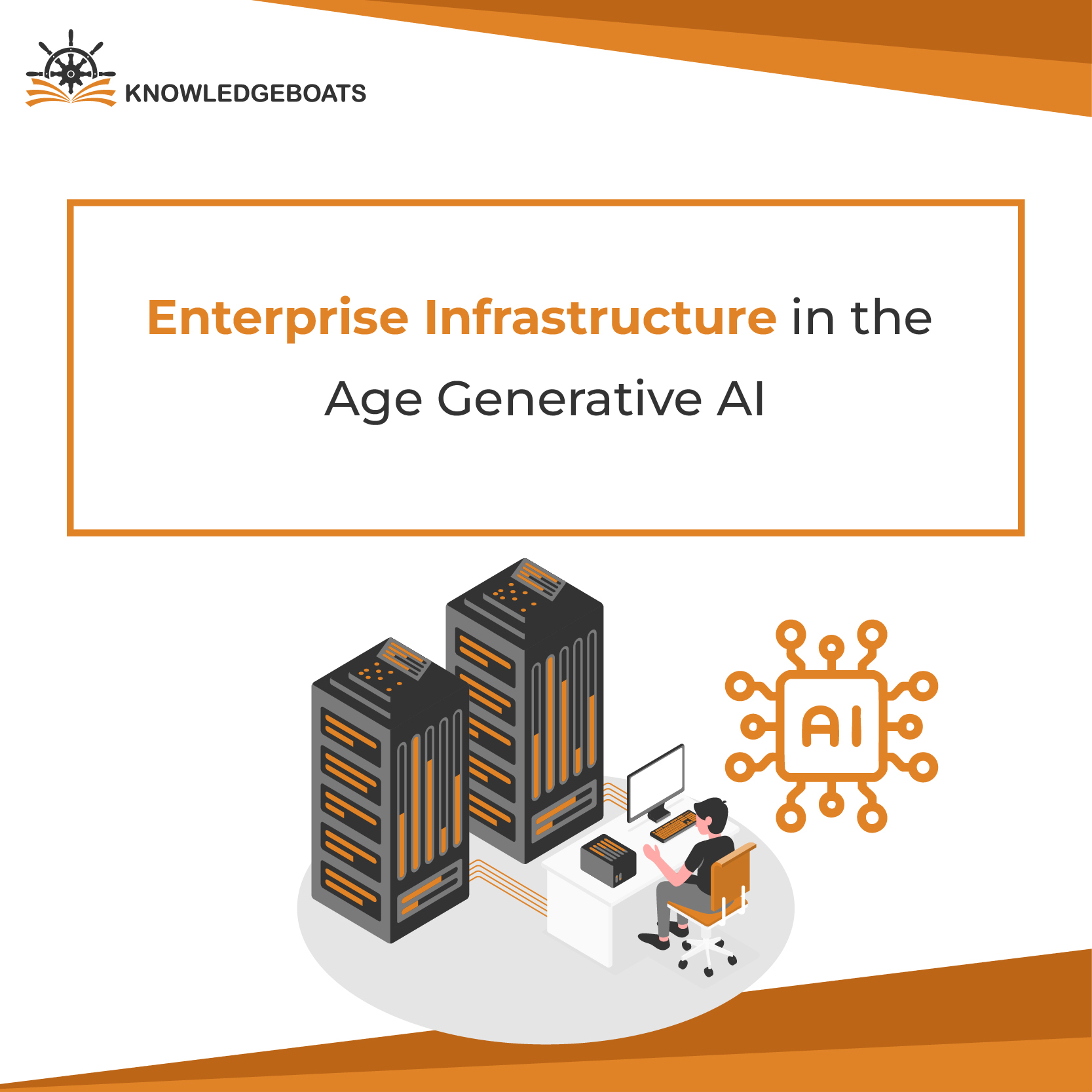
The adoption of Generative AI in different industries is increasing, captivating both professionals and the public. With a greater number of industries implementing it, many are calculating ways to expand its applications across industries. The Generative AI capabilities are clear, the challenges associated with their infrastructural needs may overwhelm the organizations.
Generative AI model deployment has swiftly transitioned from academic curiosity to business necessity from creating text, pictures, codes, and even videos. These capabilities are heavily revolutionizing the marketing, entertainment, financial, healthcare, and manufacturing sectors. Organizations deploy generative AI to help automate content creation, make decisions, and enhance customer service. Generative AI infrastructure is quickly becoming center businesses operations, from chatbots answering customer queries to AI-driven design tools producing marketing collateral.
How Generative AI Fits into Enterprise Infrastructure
Combined with enterprise architecture, Generative AI helps automate processes, validate insights, and eliminate data bottlenecks.
This allows organizations to reduce manual efforts in reporting and compliance, improve real-time decision-making and focus on delivering better customer experiences
By automating repetitive and high-level tasks, Generative AI enables leaders to redirect their attention to strategy and innovation.
Benefits of Generative AI Infrastructure in Enterprises
Generative AI infrastructure being an advanced technology helps enterprise infrastructure in various ways such as:
Improved ERP and Workflow Integration
Generative AI streamlines workflow integration through efficient links between systems like ERP, promoting fluid information transfer and real-time updates all around the process.
It streamlines workflow by utilizing the ERP systems, cured of contention and sequenced to improve operational efficiency. With the help of Generative AI human intervention is reduced, resulting in improvements in workflow management and allowing errorless data exchange.
Better Risk Management & Compliance
Generative AI assists in enhancing data validation and monitoring processes for compliance, risk management, and fraud detection by making real-time automation. It incorporates into existing workflows including routine risk assessments, ensuring compliance best practices. Generative AI infrastructure can identify anomalies and potential fraud indicators before they escalate into issues requiring manual intervention.
Forecasting Consumer Preferences
Generative AI infrastructure in enterprise architecture can anticipate consumer preferences based on the analysis of historical data so that businesses can build based on trends and customer needs. This can be embedded into several processes for personalized insight that improve real-time decision-making. Enterprises that can harness generative AI infrastructure can improve strategic foresight by taking continuous data analysis in validating consumer behavior patterns.
Optimized Maintenance and Logistics
Generative AI infrastructure improves the maintenance and logistics management for enterprise architecture by automating data analysis and predicting equipment needs or potential disruptions. This can be applied in a wide range of ways to enhance on-time maintenance and optimize logistics operations. In enterprise architecture, generative AI provides real-time monitoring of assets and supply chains, which enhances decision-making and allocation of resources.
Upgraded CRM and Operational Frameworks
Organizations need to develop their systems proactively to fulfill the demands of advanced technology. Key actions include investment in scalable cloud architecture and AI-powered swarms for productivity, along with allowing ethical AI practices.
Enhanced Data Validation and Preparation
Companies will have the ability to seamlessly scale generative AI through a concentrated focus on security, integration, data quality, and perpetual learning. As technology pivots forward, the role of helping inspire innovation, improve productivity, and open new business avenues turns it into some semblance of an imperative aspect of every enterprise landscape in future.
Key Challenges in Generative AI in enterprise implementation
There are many challenges ahead when it comes to moving from early-stage adoption to full-scale enterprise implementation of generative AI- first and foremost, enterprise infrastructure. Companies should ask themselves if their systems, processes, and tools can be scaled effectively, to allow for these new generative AI infrastructure capabilities.
One of the major concerns is creating solid data pipelines to cope with the enormous amounts of data that will make training and operation of these systems efficient. Another one is building a bridge between generative AI applications and the legacy enterprise systems. Should there be a situation where AI is not “baked into” existing enterprise systems, it can create inefficiencies, bloat, and ultimately performance-degrading errors. The absence of a centralized strategy for AI integration can inhibit adoption speed, also adding to operational costs.
Cost becomes a major shoulder by companies when it comes to scaling generative AI across an organization. These generative models have a demand of computational power that is such that high-performance computing for AI with access to powerful GPUs and possibly specialized AI chips shall come hand in hand with a requirement of ample storage to accommodate massive datasets.
Final Thoughts – AI is an Enabler, not a Replacement!
Generative AI cannot just automate your daily activities but also aid high-level decision-making. It can help in generating detailed reports, identifying trends, and provide recommendations that reduce human intervention and in-return strategic foresight.
However, it’s important to recognize that AI should complement your efforts and not aim at replacing human intelligence.
The ideal model is an intelligent collab, where humans and AI work together to intensify results.